The conducive design of technical systems requires essential preliminary considerations, particularly referring to limitations in users’ cognitive resources. These should not be overloaded due to the variety of occupied modalities and interactive features. We inspect related dynamics with multimodal behavioral experiments, computational models, and machine learning classifiers. The emerging algorithmic framework can be applied to generate adaptive assistive technologies that offer tailored support based on the detected level of cognitive load.
Multimodal cognitive load classification (2020 – 2023)
Humans’ performance varies due to different internal and external states. These states affect the amount of mental resources available to successfully pursue a task. Looking at the interplay of cognitive and affective indicators, existing research indicates the disruptive effect of emotional distractions. In particular those with negative affective valence were found to be difficult to overcome, as they might hint on potential threats. Therefore, we aim at developing reliable and robust multimodal cognitive load classifiers, which account for demands both induced by the task itself and resulting from situational and environmental influences and distractions. Since these classifications should be applicable in real-world environments, we use realistic but still controlled experimental environments to develop and test our algorithms.
Decomposing cognitive load using cognitive modeling (2016 – 2020)
To inspect cognitive dynamics underneath cognitive load in more detail, I leverage the cognitive architecture ACT-R. Its modular structure corresponds with predefined brain regions and allows to generate predictions both on the behavioral and neural level. The video below introduces my existing work in collaboration with the Cognitive Modeling Group at the University of Groningen and highlights potential future directions. It emerged within the first edition of the Mental Effort Workshop, which I co-organized until 2022.
The presented work emerged in collaboration with the Cognitive Modeling Group at the University of Groningen within the Research Training Group CrossWorlds at Chemnitz University of Technology. We thank the German Research Foundation and the Saxon State Ministry for Science, Culture and Tourism for supporting our research.
The video shows an ACT-R model performing a symbol sequence learning task with an embedded interrupting visual search task.
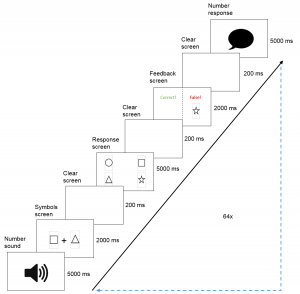
Continuous cognitive load assessment (2015 – 2018)
A prominent theory in the field of educational media, the Cognitive Load Theory, postulates different facets of cognitive load related to selected aspects of the learning situation and the learning process. Within a fruitful collaboration with the chair of Media Informatics at the TU Chemnitz, I examined the interplay between these facets in abstract learning tasks with particular focus on process-related changes across a learning task. On methodological accounts, I used a variety of approaches, ranging from experimentally obtained performance measures, paralinguistic speech indices, and psychophysiological parameters. The obtained findings indicate decreasing levels of cognitive load with increasing progress in knowledge acquisition.
Related publications
- *Lingelbach, K. F., *Gado, S., **Wirzberger, M., & **Vukelić, M. (2023). Workload-dependent hemispheric asymmetries during the emotion-cognition interaction: A close-to-naturalistic fNIRS study. Frontiers in Neuroergonomics, 4, 1273810. https://doi.org/10.3389/fnrgo.2023.1273810 *Authors share first authorship **Authors share last authorship
- *Gado, S., *Lingelbach, K., Wirzberger, M., & Vukelic, M. (2023). Decoding mental effort in a quasi-realistic scenario: A feasibility study on multimodal data fusion and classification. Sensors, 23. 6546. https://doi.org/10.3390/s23146546 *Authors share first authorship
- Williams, C. C., Weinhardt, D., Wirzberger, M., & Musslick, S. (2023). Enhancing EEG classification performance through Generative Adversarial Networks: Investigating the impact of sample sizes and classifier selection. In International Conference on Data-Integrated Simulation Science (SimTech2023). University of Stuttgart.
- *Williams, C. C., *Weinhardt, D., Wirzberger, M., & Musslick, S. (2023). Augmenting EEG with generative adversarial networks enhances brain decoding across classifiers and sample sizes. In M. Goldwater, F. K. Anggoro, B. K. Hayes, & D. C. Ong (Eds.), Proceedings of the 45th Annual Meeting of the Cognitive Science Society (pp. 1770–1776). Cognitive Science Society. [PDF] *Authors share first authorship
- Wirzberger, M. (2021). Warum Lernen (manchmal mehr, manchmal weniger) anstrengend ist [Why learning is (sometimes more, sometimes less) demanding]. Das In-Mind Magazin, 1.[ARTICLE]
- Wirzberger, M., Borst, J. P., Krems, J. F., & Rey, G. D. (2020). Memory-related cognitive load effects in an interrupted learning task: A model-based explanation. Trends in Neuroscience and Education, 20, 100139https://doi.org/10.1016/j.tine.2020.100139
- Musslick, S., Wirzberger, M., Grahek, I., Bustamante, L., Shenhav, A., & Cohen, J. D. (2020). Mental effort: One construct, many faces? In S. Denison., M. Mack, Y. Xu, & B.C. Armstrong (Eds.), 42ndAnnual Meeting of the Cognitive Science Society (pp. 1-2). Cognitive Science Society. [PDF]
- Wirzberger, M., Borst, J. P., Krems, J. F., & Rey, G. D. (2019). An ACT-R approach to investigating mechanisms of performance-related changes in an interrupted learning task. In In A. K. Goel, C. M. Seifert, & C. Freksa (Eds.) Proceedings of the 41st Annual Conference of the Cognitive Science Society (pp. 1206-1211). Montreal, QB: Cognitive Science Society. [PDF]
- Wirzberger, M., Borst, J. P., Krems, J. F., & Rey, G. D. (2019). A model-based explanation of performance related changes in abstract stimulus-response learning. In 52nd Annual Meeting of the Society for Mathematical Psychology. Program & Abstracts (pp. 19-20). Society for Mathematical Psychology.
- Wirzberger, M. (2019). Load-inducing factors in instructional design: Process-related advances in theory and assessment (Doctoral dissertation, TU Chemnitz). [PDF of synopsis part]
- Wirzberger, M., Herms, R., Esmaeili Bijarsari, S., Eibl, M., & Rey, G. D. (2018). Schema-related cognitive load influences performance, speech, and physiology in a dual-task setting: A continuous multi-measure approach. Cognitive Research: Principles and Implications, 3:46. https://doi.org/10.1186/s41235-018-0138-z
- Herms, R., Wirzberger, M., Eibl, M., & Rey, G. D. (2018). CoLoSS: Cognitive load corpus with speech and performance data from a symbol-digit dual-task. In N. Calzolari, K. Choukri, C. Cieri, T. Declerck, S. Goggi, K. Hasida, … T. Tokunaga (Eds.). Proceedings of the 11th International Language Resources and Evaluation Conference (LREC 2018) (pp. 4312-4317). Miyazaki, Japan: European Language Resources Association (ELRA). PDF
- Wirzberger, M., Herms, R., Esmaeili Bijarsari, S., Rey, G. D., & Eibl, M. (2018). Cognitive load influences performance, speech and physiological parameters in a multimodal dual-task setting. In A. C. Schütz, A. Schubö, D. Endres, & H. Lachnit (Eds.) Abstracts of the 60th Conference of Experimental Psychologists (p. 296). Lengerich: Pabst Science Publishers.
- Wirzberger, M., Herms, R., Esmaeili Bijarsari, S., Rey, G. D., & Eibl, M. (2017). Influences of cognitive load on learning performance, speech and physiological parameters in a dual-task setting. In Abstracts of the 20th Conference of the European Society for Cognitive Psychology (p. 161). Potsdam, Germany.
- Wirzberger, M., Esmaeili Bijarsari, S., & Rey, G. D. (2017). Embedded interruptions and task complexity influence schema-related cognitive load progression in an abstract learning task. Acta Psychologica, 179, 30-41. https://doi.org/10.1016/j.actpsy.2017.07.001
- Wirzberger, M., Rey, G. D., & Krems, J. F. (2017). Modeling cognitive load effects in an interrupted learning task: An ACT-R approach. In G. Gunzelmann, A. Howes, T. Tenbrink, & E. J. Davelaar (Eds.), Proceedings of the 39th Annual Meeting of the Cognitive Science Society (pp. 3540-3545). Austin, TX: Cognitive Science Society. PDF
- Wirzberger, M. (2017). Inspecting cognitive load factors in digital learning settings with ACT-R. In T. Arnold, B. Heinzerling, C. Tauchmann, T. Grube, M. Maaß, & N. Wessels (Eds.), Dagstuhl 2017. Proceedings of the 11th Joint Workshop of the German Research Training Groups in Computer Science (p. 62).
- Wirzberger, M., Beege, M., Schneider, S., Nebel, S., & Rey, G. D. (2016). One for all?! Simultaneous examination of load-inducing factors for advancing media-related instructional research. Computers & Education, 100, 18-31. https://doi.org/10.1016/j.compedu.2016.04.010
- Wirzberger, M., & Rey, G. D. (2016). Examining load-inducing factors in instructional design: An ACT-R approach. In D. Reitter, & F. E. Ritter (Eds.). Proceedings of the 14th International Conference on Cognitive Modeling (ICCM 2016) (pp. 223-224). University Park, PA: Penn State.
- Wirzberger, M., Beege, M., Schneider, S., Nebel, S., & Rey, G. D. (2016). CLT meets WMU: Simultaneous experimental manipulation of load factors in a basal working memory task. 9th International Cognitive Load Theory Conference, June 22nd to 24th, 2016, Bochum, Germany, Abstracts (p. 19).
- Wirzberger, M., Beege, M., Schneider, S., Nebel, S., & Rey, G. D. (2016). Separating cognitive load facets in a working memory updating task: An experimental approach. International Meeting of the Psychonomic Society, Granada – Spain, May 5-8, 2016, Abstract Book (pp. 211-212).
- Wirzberger, M. (2016). Modeling load factors in multimedia learning: An ACT-R approach. In B. Etzold, R. Richter, M. Eibl, & W. Lehner (Eds.). Dagstuhl 2016. Proceedings of the 10th Joint Workshop of the German Research Training Groups in Computer Science (p. 98). Chemnitz: Universitätsverlag Chemnitz.
- Wirzberger, M., & Rey, G. D. (2016). CLT meets ACT-R: Modeling load-inducing factors in instructional design. In J. Funke, J. Rummel, & A. Voß (Eds.). Abstracts of the 58th Conference of Experimental Psychologists (p. 377). Lengerich: Pabst Science Publishers.
- Wirzberger, M. & Rey, G. D. (2015). Cognitive modeling meets instructional design: Exploring Cognitive Load Theory with ACT-R. In C. Wienrich, T. Zander, & K. Gramann (Eds.). Trends in Neuroergonomics. Proceedings of the 11th Berlin Workshop Human-Machine Systems (pp. 190-193), Berlin: Universitätsverlag der TU Berlin. https://doi.org/10.14279/depositonce-4887